Effective Medical Literature Monitoring Workflows with AI
- Bruno Ohana
- Apr 5, 2022
- 4 min read
Updated: Feb 21, 2024
The monitoring of scientific literature for adverse events is a high volume activity requiring more time from specialists every day.
In this article we discuss the AI features in Biologit MLM-AI that can be integrated into screening workflows in a safe and effective way to reduce workloads and increase quality.
Literature Monitoring Screening with Prediction Tags
In MLM-AI, every search result is tagged with the prediction of different NLP and machine learning models. Each tag was developed with safety surveillance workflows in mind, for example:
In ICSR literature monitoring, it is important to detect an identifiable patient, the safety event and any special situations
For signal and aggregate reporting, aggregate studies and special situations may also be of interest
In human pharmacovigilance users may wish to exclude in-vitro studies or animal studies
The models in the Biologit MLM-AI platform were built together with safety surveillance professionals and reflect the requirements of this type of activity:
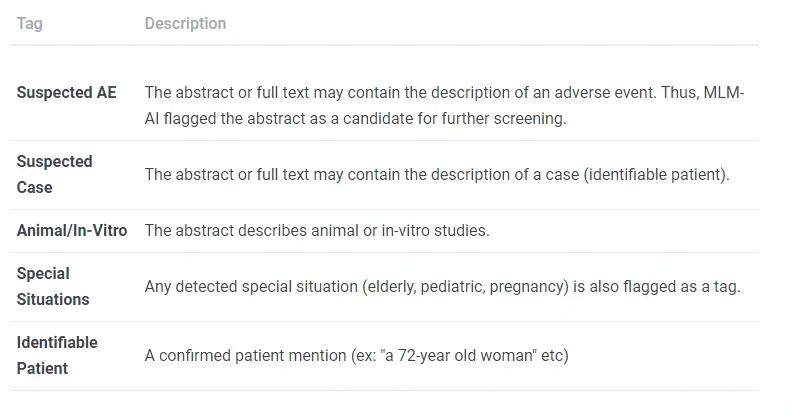
The Suspected Adverse Event model is designed to identify if the text describes a safety event (in humans), or if one may be found in the full text of the article. The main idea is to facilitate filtering articles without any safety data while ensuring important safety information is not missed - hence the prediction of a “suspected” event. The same idea of a "suspected" article is seen in other models available in MLM-AI for animal/in-vitro studies and cases.
This means a “suspected” prediction is broader than, say, a confirmed event, as illustrated in the chart below: out of all retrieved articles in a search, a subset are categorized as suspected AE, and this subset is where ICSRs (or other relevant safety-related articles) will most likely be found.
Now users can further zoom in on relevant ICSR articles with additional predictors for a suspected case, or identifiable patient.
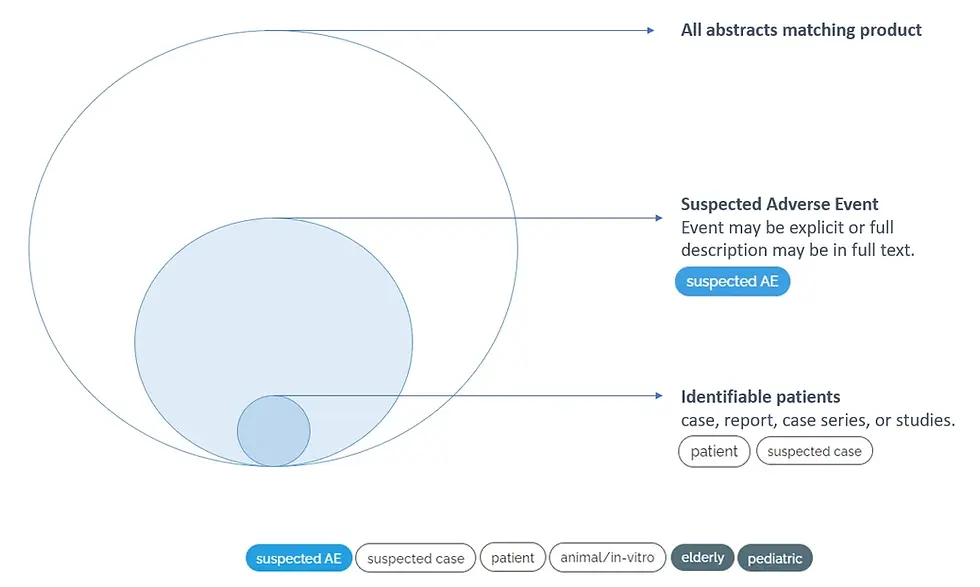
Ensuring prediction quality and AI safety
Biologit has designed the AI models and implemented governance processes following best practices that ensure models are monitored and reflect their intended use in pharmacovigilance literature workflows, for instance:
Lets see now how users of the MLM-AI platform can take advantage of these AI tags:
AI Tags for Ranking, Fast Screening and Filtering
Once results are tagged by the models, how can we use them? There are three different options that accommodate the specific levels of process oversight users may need.
Search & Rank
Tags are a useful mechanism for prioritizing the workload according to the most likely articles. For instance, in an ICSR workflow it would be useful to prioritize articles where identifiable patients are found first, followed by articles flagged as Suspected AE.
From the Review summary page, the search box allows users to craft queries taking the tags into account. Results can also be sorted according to tags. The example below filtered results according to the “Suspect AE” tag.
Once articles are reviewed, it is also possible to focus on the quality control effort where the greatest payoff is. Users can perform QC on a sample of results according to a search. For example, QC could be done on only 50% of articles not classified as Suspected AE.
"Batch" Screening
Another option is to perform a faster type of screening on articles not likely to be relevant. For instance, if the screening workflow typically excludes animal and in-vitro studies, screening these articles can be done by (1) searching by tag and (2) performing faster “batch” screening.
In batch screening abstract and title are presented in sequence according to a specified criteria and allows users to save the screening decision once across all selected articles:

Ultimate Automation: Tag Filtering
Finally, incoming results can be automatically filtered according to tags. Auto-exclude settings are applied when configuring a new search:
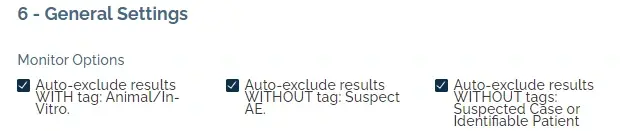
Auto-filtered results already appear as reviewed, and can still be audited/quality checked just like any other screening decision.
Safe and Auditable Processes by Design
Whatever the level of automation customers chose, MLM-AI will always present the entirety of search results and any automated decisions in an auditable activity log. Nothing is ever hidden from users. All automated decisions can undergo normal quality control processes, just as would be the case when quality checking human decisions.
Conclusions
In this post we discussed various productivity features available in biologit MLM-AI that enable more effective screening workflows in safety surveillance. Users can choose the right level of automation and process oversight that meets their requirements, all within a fully auditable environment.
AI productivity gains can save professionals time and effort. They can also lead to results of higher quality: by focusing time on results that matter, more articles can be screening leading to strategies that more comprehensively search the literature. In this blog post we used MLM-AI to assess the benefits of searching open access literature for finding new events with positive results.
About biologit MLM-AI
biologit MLM-AI is a complete literature monitoring solution built for pharmacovigilance teams. Its flexible workflow, unified scientific database, and unique AI productivity features deliver fast, inexpensive, and fully traceable results for any screening needs.
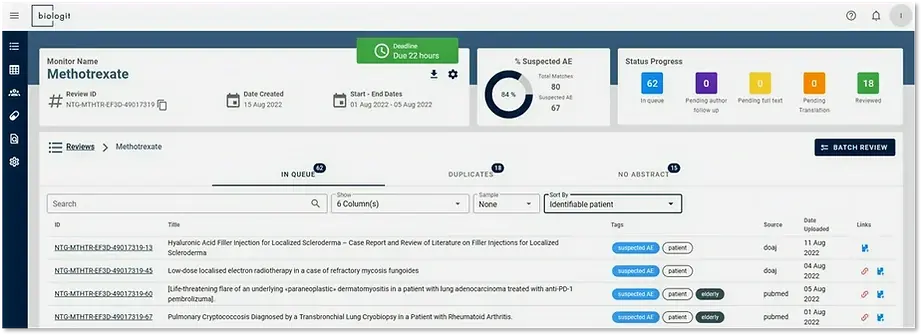
Contact us for more information on the platform and to sign up for a free trial.
Comments