Achieving faster literature screening with AI
- Bruno Ohana
- Apr 5, 2022
- 5 min read
Updated: Feb 21, 2024
Medical literature monitoring (MLM) for adverse drug reactions is an important aspect of the pharmacovigilance process and a regulatory requirement for medicinal products on the market.
Today, this is a high volume task demanding significant time from pharmacovigilance specialists. This effort however yields a small payoff: only a fraction of articles screened tend to become a valid individual case safety reports (ICSRs) of relevance to authorization holders. In addition, the volume of real world data companies are expected to review continues to grow fast: by some estimates, literature output grows by about 8% each year.
Artificial intelligence in medical literature screening
These challenges present clear opportunities for automating workflows. In particular artificial intelligence and natural language processing technologies (NLP) can play an important role.
For example: AI can be used to prioritize retrieved articles so the most important and potentially serious ones are reviewed first; NLP techniques that identify relevant sections of text can be employed to facilitate screening and save reviewer time highlighting important aspects of the text (medication or patient mentions, for example).
While important, these uses of AI still fall short of the true potential of this technology. The continued growth in the volume of scientific literature demands re-framing the use of AI in medical literature monitoring tasks so that companies can search more broadly, find more events faster and allow pharmacovigilance specialists to perform other high value activities.
We ask: can we apply recent advances in machine learning and NLP to effectively filter irrelevant articles from the screening process, without impacting results quality?
Using AI as a filter means going beyond incremental improvements that still require specialists to review every incoming article towards effectively decoupling human effort from the volume of incoming articles (recall the rate of ICSRs from search hits is very small).
The diagram below shows a simplified medical literature monitoring process with an AI filter deployed to remove irrelevant articles in the early, high-volume stages of the workflow before articles are seen by a specialist screener:

AI as a pre-screening filter of article abstracts
Our aim is to reduce the volume of incoming abstracts before they get the first review by a specialist. At this point, we are faced with important questions on how to design our AI system:
Abstracts contain incomplete information: adverse reactions may only be implied and only fully described in the full article.
Similarly, determining causality of an event to a product of interest may also require confirmation by reading the full article.
Even with these limitations, it may still be possible to filter irrelevant articles that contain no evidence an adverse event is stated or implied. In fact, this is precisely the objective of a specialist that performs abstract screening, which AI models should emulate. In other words, we wish to train models that look for evidence of a suspected adverse event, which we define as:
when an adverse drug reaction is described explicitly in the abstract or title, or is implied (in which case it is further detailed in the full article).
event causality to any particular drug of interest is not taken into account: a suspect adverse event may be linked to any drug or treatment described in the abstract.
Clearly, an article flagged as suspected adverse event can still turn out not to be an ICSR for a certain product. This approach however mitigates the risk of missing a real event: any ICSR should also be a suspected adverse event in the first place, and has the additional benefit of producing more robust drug-agnostic models. This is the approach we take to train models for the biologit MLM-AI solution.
With help from our team of pharmacovigilance experts, we prepared a dataset of abstracts from biomedical literature covering a broad range of products, and created labeling guidelines following the above criteria. To assess performance, we used this dataset to a deep learning model similar to previous good benchmarks in NLP tasks, and the SciSpacy pre-trained embeddings, which use biomedical text as source.
To evaluate results, it would be great to avail of a gold standard and independently verified dataset of screening results. Even better if this dataset comprised multiple products. Fortunately, the EMA performs literature screening on a number of products and regularly issues screening results. We are thankful to the EMA for sharing a dataset with us for our evaluation.
Once models were trained, we used them to predict articles as suspected adverse on the EMA dataset (after removing any examples that was also present in our dataset to prevent contamination of results). The experiment is further detailed in our recently published study in the DIA regulatory science forum.
Simulating safe thresholds levels
In literature screening, it is important to avoid missing out on potential events. The AI models should retrieve almost all articles that are an ICSR candidates, while still saving time by filtering irrelevant ones. To simulate this outcome, we evaluate the volume of irrelevant articles filtered for a target recall of 95%. Other values of recall can be considered depending on the needs of particular MLM processes.
We can calibrate our model to issue predictions for the desired recall using historical data. The chart below shows the trend of % articles saved vs. desired recall, for each month tested (calibrated using data from previous month).
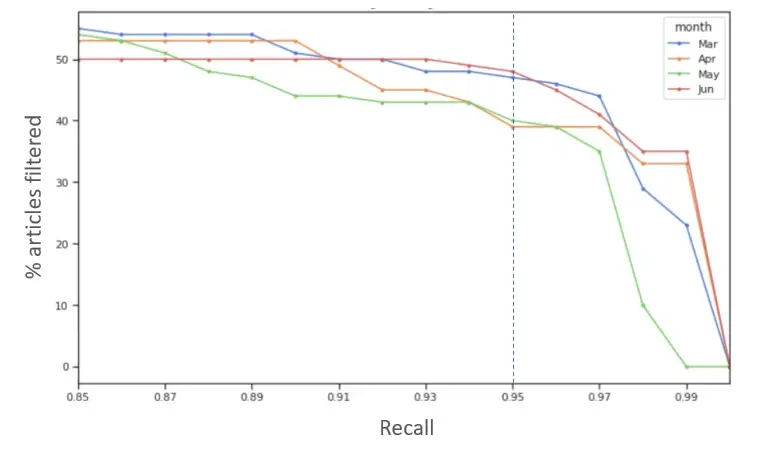
Results from our experiment indicated calibration of the prediction thresholds to maintain a target recall worked well, and filtering achieved savings in excess of 40% (up to 49%):
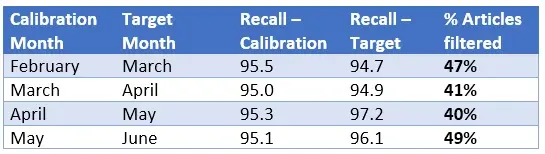
Next steps
Our results applied a benchmark AI model trained using our proposed suspect adverse event approach. Preliminary results using benchmark models and a small sized training dataset have shown promising savings, filtering in excess of 40% of inbound articles while maintaining 95% recall.
We are constantly refining our models and data labeling efforts based on feedback from our experimentation. As labeling data continues to grow and by refining AI models to this particular task we believe further gains can be obtained while maintaining high levels of quality in results.
Lastly, we know safe and trustworthy AI in the drug safety is paramount in the life sciences industry: we continue to monitor and evolve our approach in light of upcoming regulatory guidance for pharmacovigilance.
Learn more
Learn more about biologit MLM-AI, our AI-powered MLM solution built from the ground up for pharmacovigilance workflows.
For more details on AI models shipped in MLM-AI, check out our post about model fact sheets.
See our recent article published on DIA 2020 Regulatory Science forum.
About Biologit MLM-AI
biologit MLM-AI is a complete literature monitoring solution built for pharmacovigilance teams. Its flexible workflow, unified scientific database, and unique AI productivity features deliver fast, inexpensive, and fully traceable results for any screening needs.
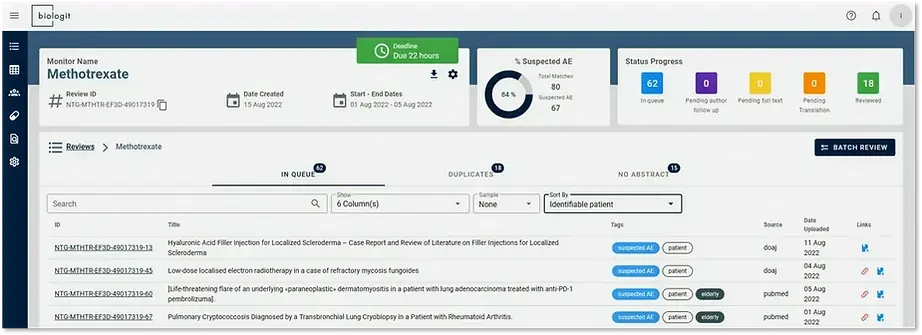
Comments